Why Ask People What They Think About Climate Change When Chat-GPT Can Just Be Racist and Wrong About It?
Photo by Justin Sullivan/Getty Images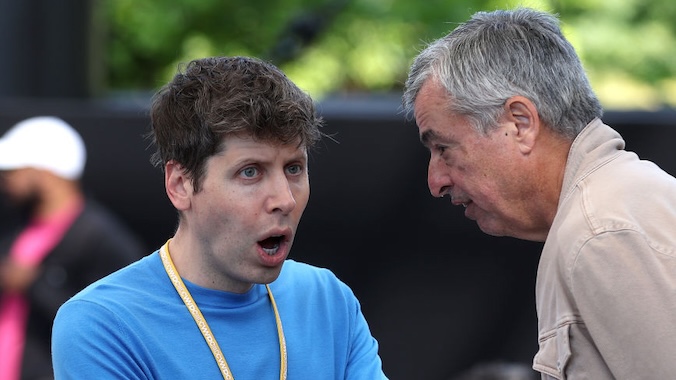
Opinion polling is tough these days. Who answers the phone anymore, anyway, especially from an unknown number? Why would you click that link from something apparently named after video game laser sounds? And if you do respond to polling, why not mess around with it, just for fun? It’s not an election or anything.
But polling is important (probably?), both for understanding politics and elections and also for having a solid grasp on where the populace stands on issues ranging from gun control and immigration to abortion and the economy. Thus, a burgeoning field of research has set out to ask the important question of whether the now-ubiquitous plagiarism machines can take over for the humans.
“LLMs [large language models] have demonstrated significant potential for contributing to social science research,” wrote authors of a new study published Wednesday in PLoS Climate. “A recent development lies in their capacity to accurately replicate the perceptions, viewpoints, and behavior of the general population.”
Sounds impressive! LLMs like GPT able to answer polling questions that humans would prefer to ignore, and in a way that accurately reflects what those humans really think? What a boon! The researchers in the new study turned the LLM’s considerable pseudo-intellect toward global warming opinion, to see if they could replicate what existing surveys have told us already.
Spoiler, the LLMs are racist and often wrong.
“GPT-4 underestimated Non-Hispanic Blacks believing that global warming is happening,” the authors found. In fact, Black Americans have shown generally higher levels of concern over climate change than other demographic groups; the disparity with GPT’s guesses, though, is not a shock. “LLMs often reflect the biases inherent in their training data, which can lead to biased outputs,” the authors continued. “These biases are particularly pronounced when considering groups that are underrepresented in the data sets.”
Overall, when comparing GPT-3.5 and GPT-4 with actual survey results from 2017 and 2021, the LLMs answered a question about belief that global warming is happening correctly 85 percent of the time — not bad, if you forget about the humans who answered it “correctly” 100 percent of the time.
Sometimes they fared worse. If conditioned only on demographic information of the people they are trying to emulate, the LLMs sometimes dramatically overestimated Americans’ beliefs.
“Interestingly, these models seem to assume a universal belief in global warming, an assumption that does not accurately reflect the diversity of real-world viewpoints in the U.S.,” the authors wrote, apparently without irony. When other variables such as “awareness of the scientific consensus” were added to the model, the accuracy improved. Somewhat.
But add even more complexity and they get worse again: Instead of just a yes/no question about warming’s existence, when GPT was faced with an additional “I don’t know” possibility its accuracy declined from a maximum of 85 percent to 75 percent.
Even if we accept the fundamental “why” at the root of such research — there are gaps in polling and good LLMs might fill them, offering a better understanding of public opinion and societal priorities — the glaring limitations of the “artificial intelligence” continually being shoved down our throats suggests we might be putting the cart before the horse here. Like amphibian DNA upending a perfectly good theme park’s plans, using half-baked techno-hallucinations to fill in the gaps of human opinion seems rife for error and abuse.
To be fair, the authors of the new paper insist that LLMs aren’t going to simply replace polling. “[W]e propose employing LLMs as a complementary instrument for preliminary investigations, survey design, outcome forecasting, and hypothesis generation,” they write. Only, the temptation to cut corners and cut costs could end up too great — the researchers said their “synthetic samples” came at a far lower cost than traditional survey methods offer, as low as around two bucks per “person” sampled.
Outsourcing human activities to nebulous AI systems (the authors of the new paper also decried OpenAI’s utter lack of transparency on how their LLMs actually work) has already proven to have potentially ugly consequences, even while those bad stories can obscure some truly transformational things an LLM or similar tool can do. There may yet be positive and useful outcomes to it, but adding “human opinions” to the things AI is capable of replicating, whether on global warming or any other topic, is at this point a tough pill to swallow.